对象存储密度低
Full GC 影响性能
OOM 影响稳定
Cache Miss
- 基础数据结构(package:org.apache.flink.core.memory)
- 内存管理机制(package:org.apache.flink.runtime.memory)
Flink 中的 Worker 名叫 TaskManager,是用来运行用户代码的 JVM 进程。
入口:
TaskManagerRunner.main() --> runTaskManager() --> ... -->TaskManagerServices
1
2
3
4
5
6
7
8
9
10
11
12
| public class TaskManagerServices {
...
private final MemoryManager memoryManager;
private final NetworkEnvironment networkEnvironment;
...
public static TaskManagerServices fromConfiguration() {
...
final NetworkEnvironment network = createNetworkEnvironment(taskManagerServicesConfiguration, maxJvmHeapMemory);
final MemoryManager memoryManager = createMemoryManager(taskManagerServicesConfiguration, freeHeapMemoryWithDefrag, maxJvmHeapMemory);
...
}
}
|
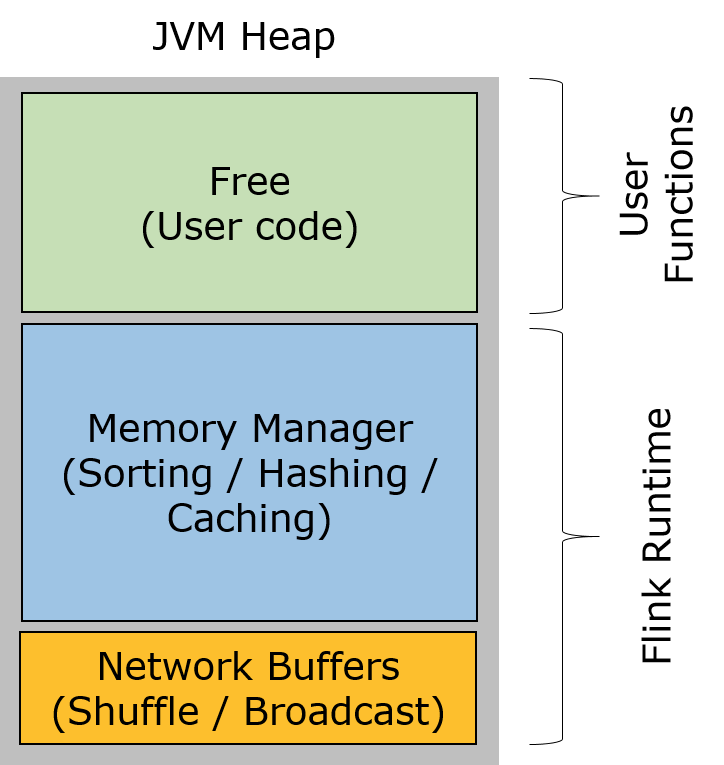
- Network Buffers: 一定数量的32KB大小的 buffer,主要用于数据的网络传输。在 TaskManager 启动的时候就会分配。默认数量是 2048 个,可以通过
taskmanager.network.numberOfBuffers
来配置。 - Memory Manager Pool: 这是一个由
MemoryManager
管理的,由众多MemorySegment
组成的超大集合。Flink 中的算法(如 sort/shuffle/join)会向这个内存池申请 MemorySegment,将序列化后的数据存于其中,使用完后释放回内存池。默认情况下,池子占了堆内存的 70% 的大小。 - Remaining (Free) Heap: 这部分的内存是留给用户代码以及 TaskManager 的数据结构使用的。因为这些数据结构一般都很小,所以基本上这些内存都是给用户代码使用的。从GC的角度来看,可以把这里看成的新生代,也就是说这里主要都是由用户代码生成的短期对象。
基础的内存数据结构:
内存管理器:MemoryManager
内存池: MemoryPool/HybridHeapMemoryPool/HybridOffHeapMemoryPool
内存片段:MemorySegment/HeapMemorySegment/HybridMemorySegment
数据视图:DataInputView/DataOutputView
内存管理器(MemoryManager)
1
2
3
4
5
6
7
8
9
10
11
12
13
14
15
16
17
18
19
20
21
22
23
24
25
26
27
28
| public class MemoryManager {
private final MemoryPool memoryPool; //内存池
private final MemoryType memoryType; //内存类型,HEAP/OFF_HEAP
private final boolean isPreAllocated; //是否预先分配
private final HashMap<Object, Set<MemorySegment>> allocatedSegments; //已分配的内存片段
public MemoryManager(...) {
...
switch (memoryType) {
case HEAP:
this.memoryPool = new HybridHeapMemoryPool(memToAllocate, pageSize);
break;
case OFF_HEAP:
if (!preAllocateMemory) {
LOG.warn("It is advisable to set 'taskmanager.memory.preallocate' to true when" +
" the memory type 'taskmanager.memory.off-heap' is set to true.");
}
this.memoryPool = new HybridOffHeapMemoryPool(memToAllocate, pageSize);
break;
default:
throw new IllegalArgumentException("unrecognized memory type: " + memoryType);
}
}
public void allocatePages(Object owner, List<MemorySegment> target, int numPages);
public void release(MemorySegment segment);
public void releaseAll(Object owner);
}
|
1
2
3
4
5
6
7
8
9
10
11
12
13
14
15
16
17
18
19
20
21
22
23
24
25
26
27
| abstract static class MemoryPool {
abstract int getNumberOfAvailableMemorySegments(); //获取有效内存片段数
abstract MemorySegment allocateNewSegment(Object owner); //分配新的内存片段
abstract MemorySegment requestSegmentFromPool(Object owner); //从内存池中获取内存片段
abstract void returnSegmentToPool(MemorySegment segment); //将内存片段还给内存池
abstract void clear();
}
HybridHeapMemoryPool(int numInitialSegments, int segmentSize) {
this.availableMemory = new ArrayDeque<>(numInitialSegments);
this.segmentSize = segmentSize;
for (int i = 0; i < numInitialSegments; i++) {
// 使用 new 在 jvm 堆上分配内存
this.availableMemory.add(new byte[segmentSize]);
}
}
HybridOffHeapMemoryPool(int numInitialSegments, int segmentSize) {
this.availableMemory = new ArrayDeque<>(numInitialSegments);
this.segmentSize = segmentSize;
for (int i = 0; i < numInitialSegments; i++) {
// 使用 allocateDirect 直接堆外内存
this.availableMemory.add(ByteBuffer.allocateDirect(segmentSize));
}
}
|
1
2
3
4
5
6
7
8
9
10
11
12
13
14
15
| public final class MemorySegmentFactory {
...
// 从池外(jvm堆中)分配内存段
public static MemorySegment allocateUnpooledSegment(int size, Object owner) {
return new HybridMemorySegment(new byte[size], owner);
}
// 从内存池 中 获取堆内存片段
public static MemorySegment wrapPooledHeapMemory(byte[] memory, Object owner) {
return new HybridMemorySegment(memory, owner);
}
// 从内存池中 获取 非堆内存片段
public static MemorySegment wrapPooledOffHeapMemory(ByteBuffer memory, Object owner) {
return new HybridMemorySegment(memory, owner);
}
}
|
1
2
3
4
5
6
7
8
9
10
11
| public abstract class MemorySegment {
protected final byte[] heapMemory; //堆内存
protected long address; //字节数组对应的相对地址(若heapMemory为null,即可能为off-heap内存的绝对地址
protected final long addressLimit; //
protected static final sun.misc.Unsafe UNSAFE = MemoryUtils.UNSAFE; //用来对堆/非堆内存进行操作,是JVM的非安全的API
protected static final long BYTE_ARRAY_BASE_OFFSET = UNSAFE.arrayBaseOffset(byte[].class); //二进制字节数组的起始索引,相对于字节数组对象
private static final boolean LITTLE_ENDIAN = (ByteOrder.nativeOrder() == ByteOrder.LITTLE_ENDIAN); //字节序
protected final int size; //内存段的字节数
private final Object owner; //该内存段owner
...
}
|
1
2
3
4
5
6
7
8
9
10
11
| //堆内存
public final class HeapMemorySegment extends MemorySegment {
// 指向heapMemory的额外引用,用来如数组越界的检查
private byte[] memory;
// 只能初始化堆内存
HeapMemorySegment(byte[] memory, Object owner) {
super(Objects.requireNonNull(memory), owner);
this.memory = memory;
}
//...
}
|
1
2
3
4
5
6
7
8
9
10
11
12
13
14
15
| //混合内存
public final class HybridMemorySegment extends MemorySegment {
private final ByteBuffer offHeapBuffer;
// 非堆内存
HybridMemorySegment(ByteBuffer buffer, Object owner) {
super(checkBufferAndGetAddress(buffer), buffer.capacity(), owner);
this.offHeapBuffer = buffer;
}
// 堆内存初始化
HybridMemorySegment(byte[] buffer, Object owner) {
super(buffer, owner);
this.offHeapBuffer = null;
}
|
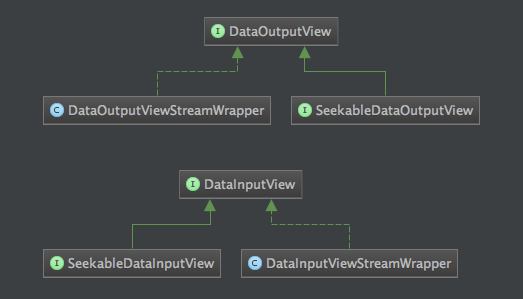
提供内存逻辑视图操作
1
2
3
4
5
6
7
8
9
10
| public interface DataInputView extends DataInput {
void skipBytesToRead(int numBytes) throws IOException;
int read(byte[] b, int off, int len) throws IOException; //读取数据到b中
int read(byte[] b) throws IOException;
}
public interface DataOutputView extends DataOutput {
void skipBytesToWrite(int numBytes) throws IOException;
void write(DataInputView source, int numBytes) throws IOException; //
}
|
Flink 中处理的数据流通常是同一类型,由于数据集对象的类型固定,对于数据集可以只保存一份对象Schema信息,节省大量的存储空间。同时,对于固定大小的类型,也可通过固定的偏移位置存取。当我们需要访问某个对象成员变量的时候,通过定制的序列化工具,并不需要反序列化整个Java对象,而是可以直接通过偏移量,只是反序列化特定的对象成员变量。如果对象的成员变量较多时,能够大大减少Java对象的创建开销,以及内存数据的拷贝大小。
Flink支持任意的Java或是Scala类型。Flink 在数据类型上有很大的进步,不需要实现一个特定的接口(像Hadoop中的org.apache.hadoop.io.Writable
),Flink 能够自动识别数据类型。Flink 通过 Java Reflection 框架分析基于 Java 的 Flink 程序 UDF (User Define Function)的返回类型的类型信息,通过 Scala Compiler 分析基于 Scala 的 Flink 程序 UDF 的返回类型的类型信息。类型信息由 TypeInformation
类表示,TypeInformation 支持以下几种类型:
BasicTypeInfo
: 任意Java 基本类型(装箱的)或 String 类型。BasicArrayTypeInfo
: 任意Java基本类型数组(装箱的)或 String 数组。WritableTypeInfo
: 任意 Hadoop Writable 接口的实现类。TupleTypeInfo
: 任意的 Flink Tuple 类型(支持Tuple1 to Tuple25)。Flink tuples 是固定长度固定类型的Java Tuple实现。CaseClassTypeInfo
: 任意的 Scala CaseClass(包括 Scala tuples)。PojoTypeInfo
: 任意的 POJO (Java or Scala),例如,Java对象的所有成员变量,要么是 public 修饰符定义,要么有 getter/setter 方法。GenericTypeInfo
: 任意无法匹配之前几种类型的类。
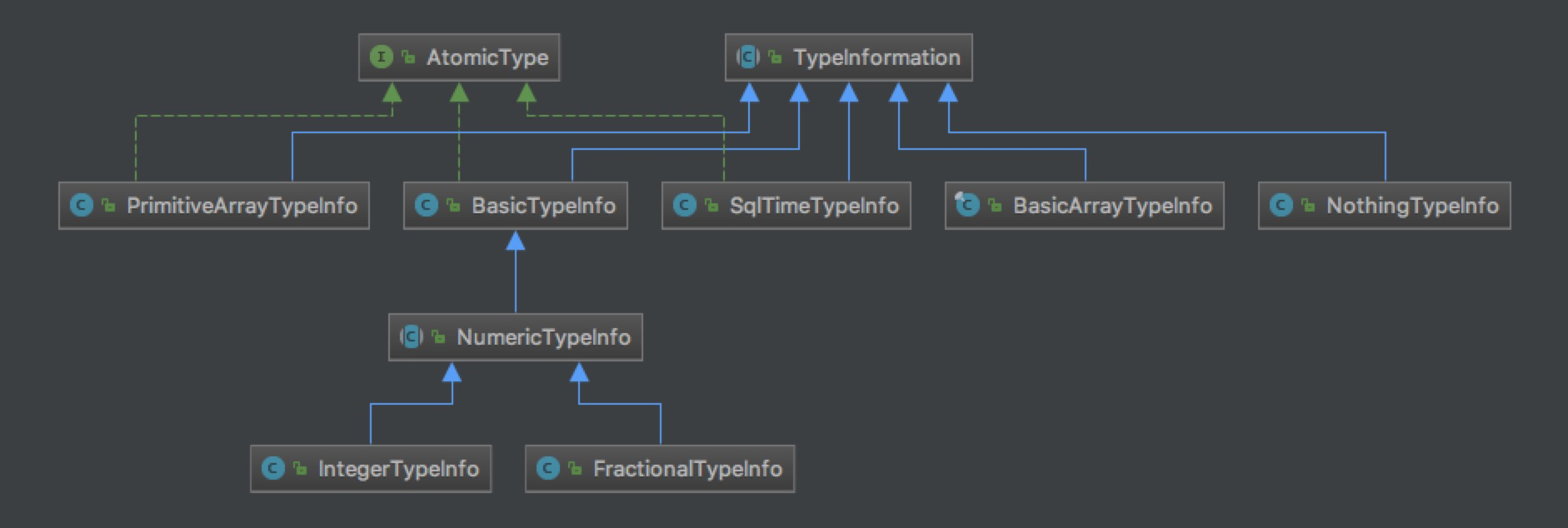
如下图展示 一个内嵌型的Tuple3 对象的序列化过程。
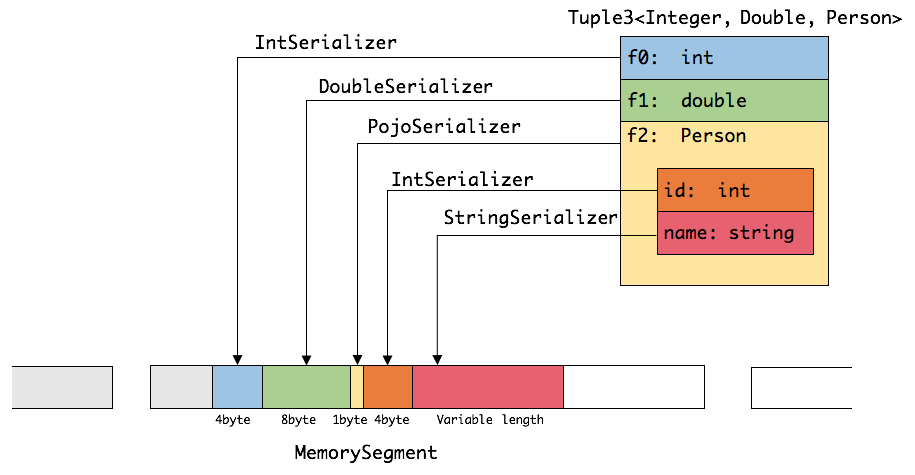
可以看出这种序列化方式存储密度是相当紧凑的。其中 int 占4字节,double 占8字节,POJO多个一个字节的header,PojoSerializer只负责将header序列化进去,并委托每个字段对应的serializer对字段进行序列化。
Flink 的类型系统可以很轻松地扩展出自定义的TypeInformation、Serializer以及Comparator,来提升数据类型在序列化和比较时的性能。
Flink 提供了如 group、sort、join 等操作,这些操作都需要访问海量数据。这里,我们以sort为例,这是一个在 Flink 中使用非常频繁的操作。
首先,Flink 会从 MemoryManager 中申请一批 MemorySegment,我们把这批 MemorySegment 称作 sort buffer,用来存放排序的数据。
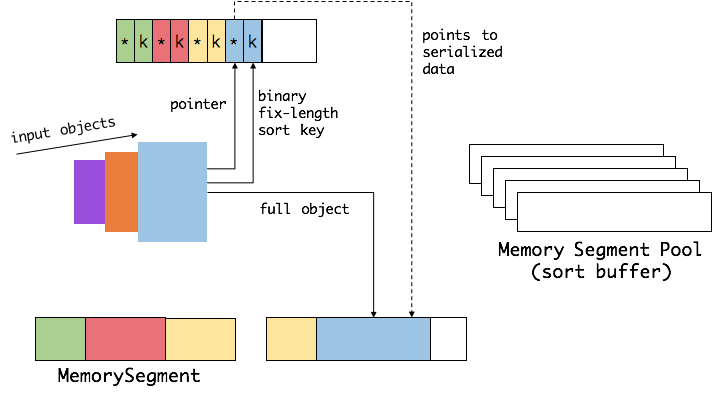
我们会把 sort buffer 分成两块区域。一个区域是用来存放所有对象完整的二进制数据。另一个区域用来存放指向完整二进制数据的指针以及定长的序列化后的key(key+pointer)。如果需要序列化的key是个变长类型,如String,则会取其前缀序列化。如上图所示,当一个对象要加到 sort buffer 中时,它的二进制数据会被加到第一个区域,指针(可能还有key)会被加到第二个区域。
将实际的数据和指针加定长key分开存放有两个目的:
第一,交换定长块(key+pointer)更高效,不用交换真实的数据也不用移动其他key和pointer。
第二,这样做是缓存友好的,因为key都是连续存储在内存中的,可以大大减少 cache miss。
排序的关键是比大小和交换。Flink 中,会先用 key 比大小,这样就可以直接用二进制的key比较而不需要反序列化出整个对象。因为key是定长的,所以如果key相同(或者没有提供二进制key),那就必须将真实的二进制数据反序列化出来,然后再做比较。之后,只需要交换key+pointer就可以达到排序的效果,真实的数据不用移动。
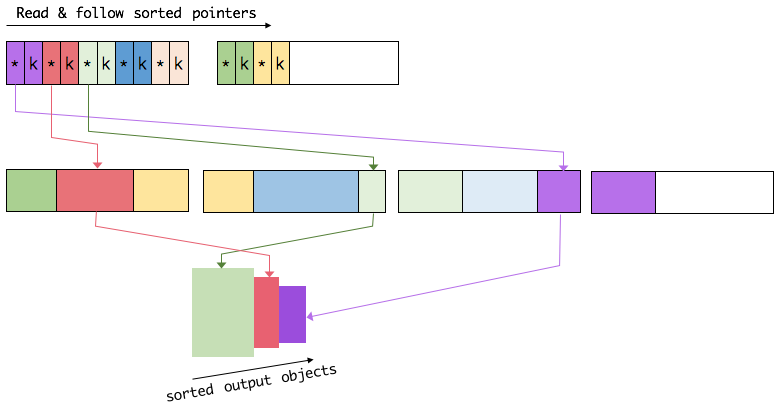
- 节省内存空间
- 高效的内存操作
- 缓存友好的计算
- 减少OOM
- 减少GC
- https://juejin.im/post/5c4f16dbe51d454f342fb7e7